When it comes to data we are more or less lost nowadays. We can acquire more and more data for a current area and find answers on our questions. The Principal Component Analysis (PCA) can help you to enhance your understanding your data and to reveal underlying information that influences your data fundamentally. Since some days there is a special plugin for QGIS available that enables you to determine principal components from your data.
the data and the plugin
For a PCA you will need multidimensional data. Looking at the plugin, you will need a layer stack in a tiff file. You can start easily and download some Landsat layers at landcover.org. Just create a layer stack using the merge function. Now you need to install the plugin by choosing plugins->manage and install plugins and search for the plugin PCA. Unfortunately the description of the plugin is more then sparse:
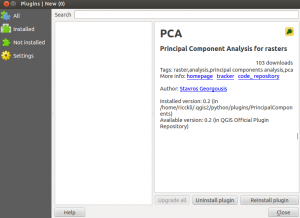
the doing
The plugin is quite simple and therefore limited in its possibilities. The plugin just needs the layer stack as input and the number of principal components. A principal component is a vector through the n-dimensional space that tries to fill most space. Maybe this image explains it:
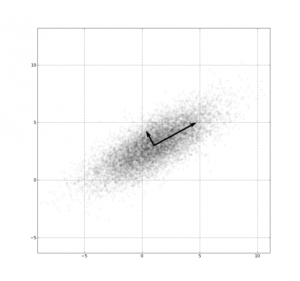
So when it comes to our input data: what underlying PCs do we have. The input data consists only of numbers that are representing the reflectance in the certain bands.
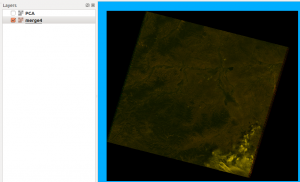
The Output is a raster with the number of PCs as bands:
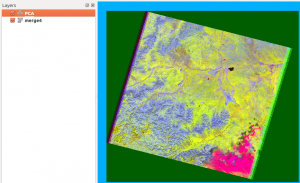
Oh, that looks flashy.
the results
The results of the plugin is a raster file containing the coordinates regarding the PCs in a layer stack. Second output is a .txt file containing the basic statistics behind the data. Especially the Eigenvalues of the PCs are important to determine the quality of the model. In our short test data set we can clearly see, that the PCs contains information of water (black), and vegetation (blue):
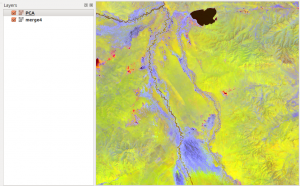
The export file is very easy to read:

Thank you Stavros Georgousis for this nice plugin. Please add rotation of PCs in the next releases. And unfortunately it takes quite a long time for my data: 16.000.000 pixels in 6 dimensions.
caution
The example above was just a quick shot!!! You need to preprocess your dataset a lot to get good and reliable results. I recommend the following papers for using remote sensed data for a PCA:
Klinger, R., Schwanghart, W. Schütt, B. (2011): Landscape classification using principal component analysis and fuzzy classification: Archaeological sites and their natural surroundings in Central Mongolia. Die Erde, 142(3), 213-233.  pdf
L. EKLUNDH, A. SINGH (1993): A comparative analysis of standardised and unstandardised Principal Components Analysis in remote sensing. International Journal of Remote Sensing Vol. 14, Iss. 7, 1993. pdf
A. Bateson, B. Curtiss (1996): A method for manual endmember selection and spectral unmixing, Remote Sensing of Environment, Volume 55, Issue 3, March 1996, Pages 229-243. pdf
Hi, my name anggoro from Indonesia. I still confused to make vegetation density using PCA from Advanced vegetation index (AVI) and Bare Soil Index (BI). Is it possible to have 2 raster file for input?